Blog
Explore a wide range of topics, get inspired by the latest trends, and learn how to create conversational experiences that make an impact on your bottom line.
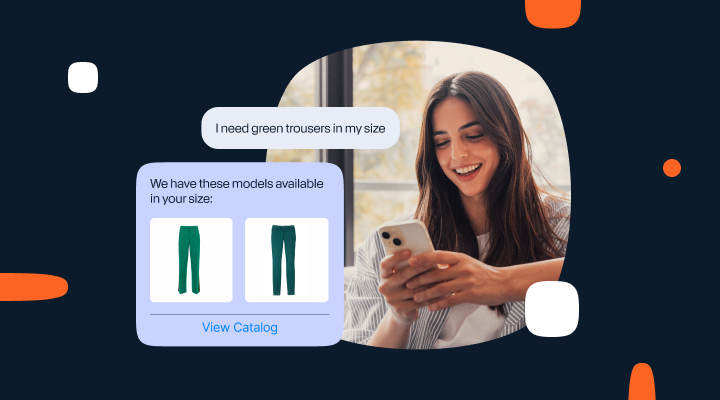
July 25, 2025
Conversational commerce in retail
What is conversational commerce in retail? How can retailers capitalize on it and what are the benefits? Find out this and more!
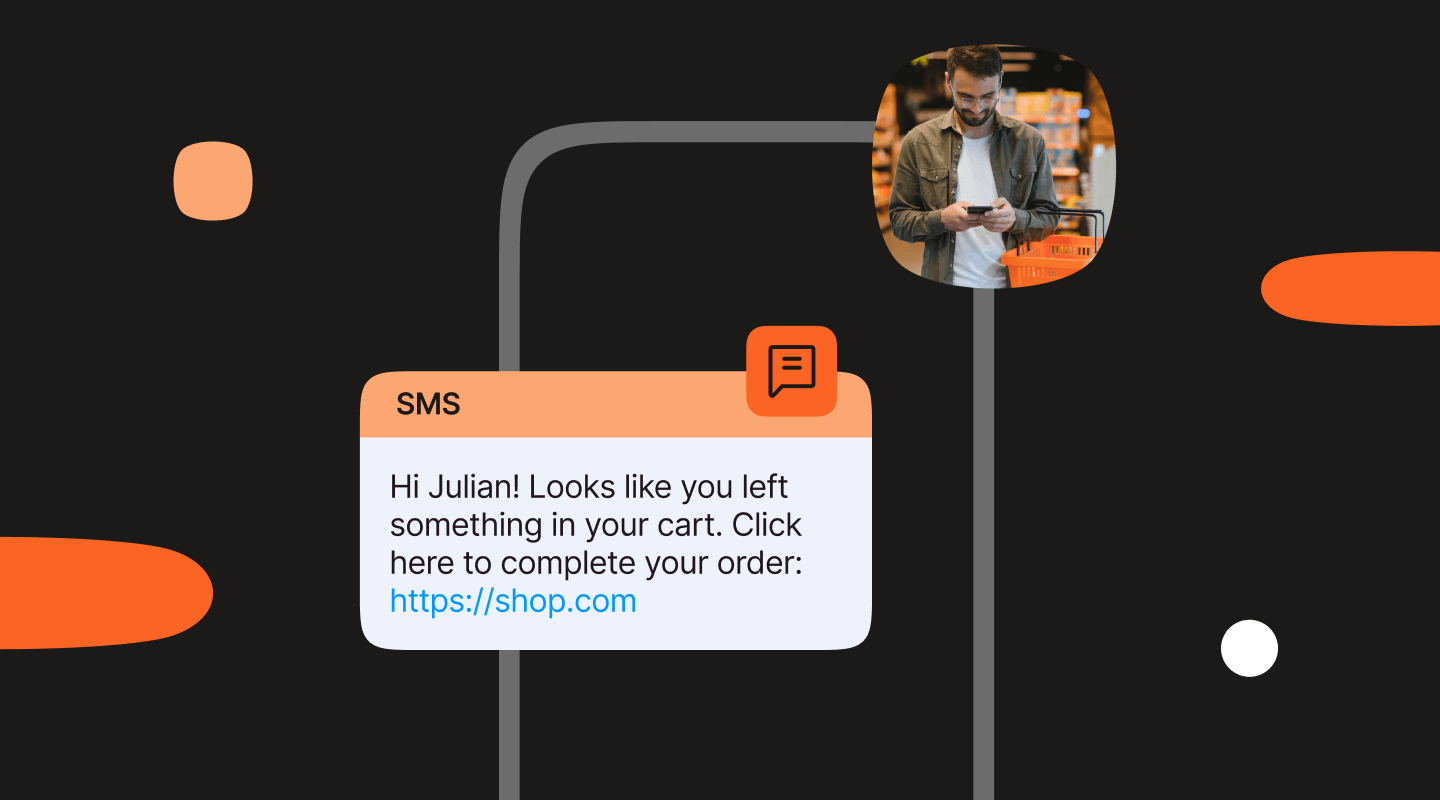
July 25, 2025
How to schedule a text message: A step-by-step guide to timing your SMS perfectly
Discover three easy ways to schedule your text messages with Infobip. With no code options to SMS API, there is a solution for everyone.
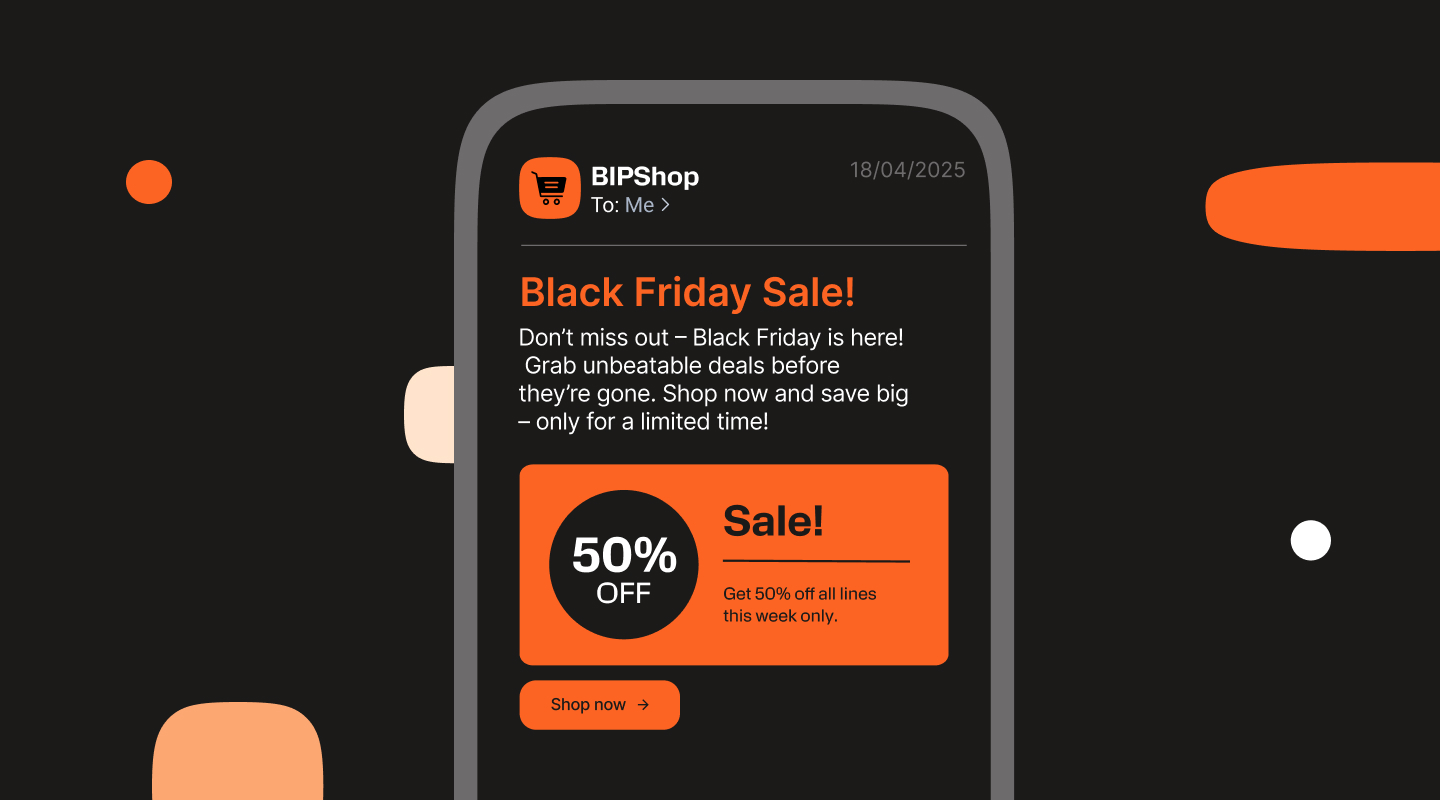
July 24, 2025
How to nail your email delivery strategy ahead of Black Friday and the shopping season
With the peak shopping season now extending from October to January, now is the time to ensure that your email strategy and infrastructure is ready to capitalize. Find out how with our detailed guide.
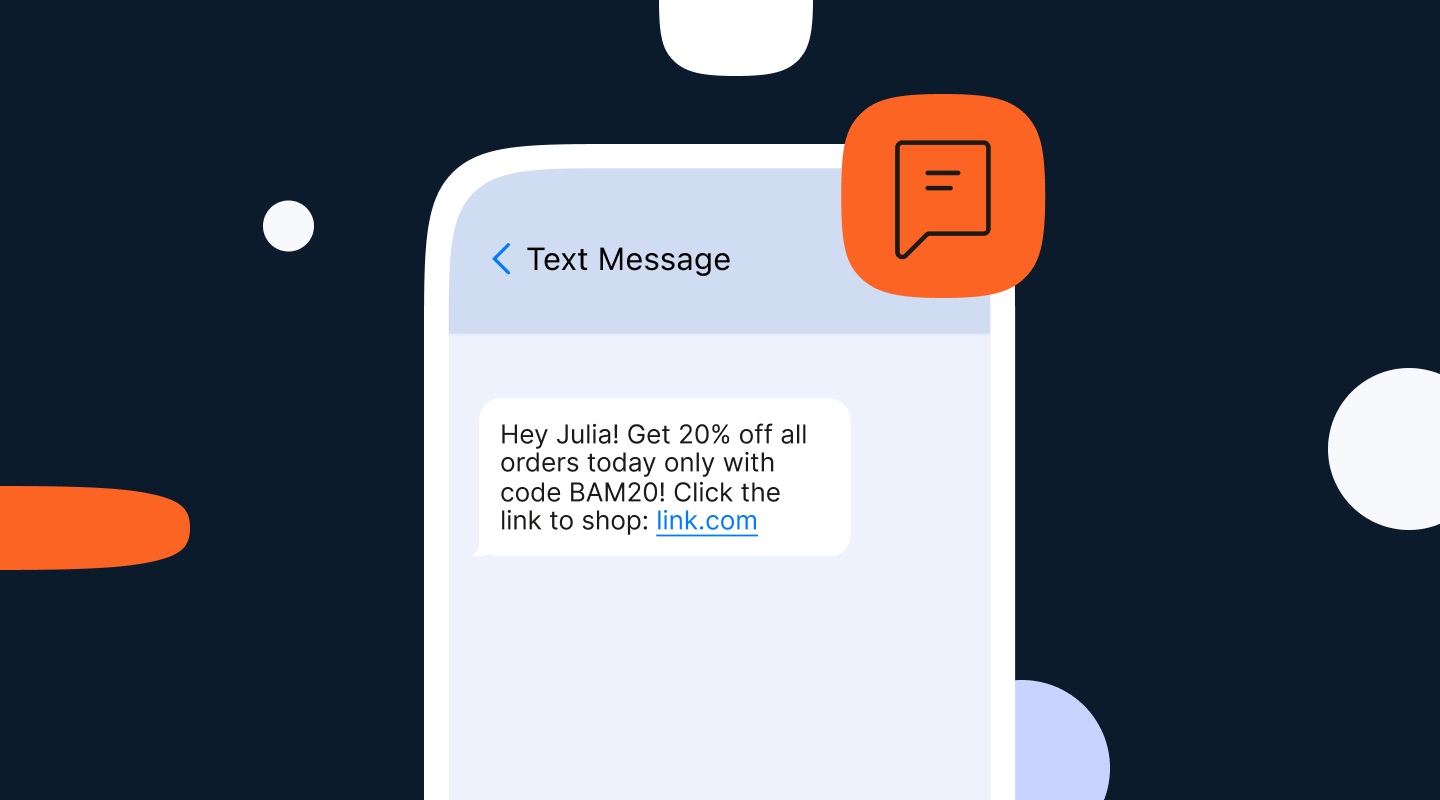
July 24, 2025
Personalized text messages: Tips, examples, and best practices
Discover how businesses can use personalized SMS to deliver timely, relevant messages that improve customer engagement and loyalty.
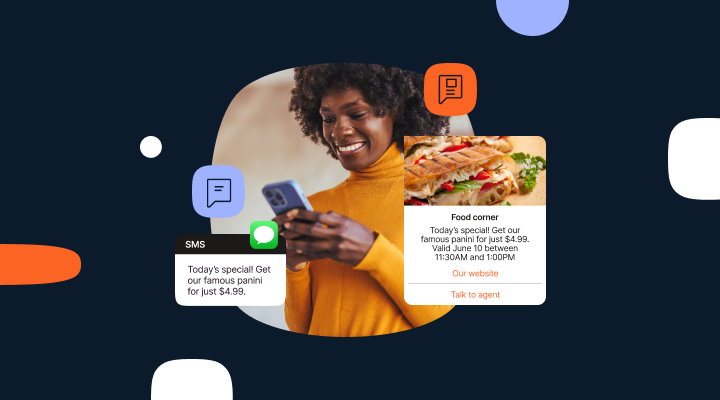
July 23, 2025
RCS vs SMS: Which is best for your business?
Let’s look closely at RCS, how brands can benefit from its unique features, and how it compares to SMS overall as a business messaging tool. We go over use cases where businesses have been able to combine SMS and RCS effectively to provide a better and more cost-effective customer experience.
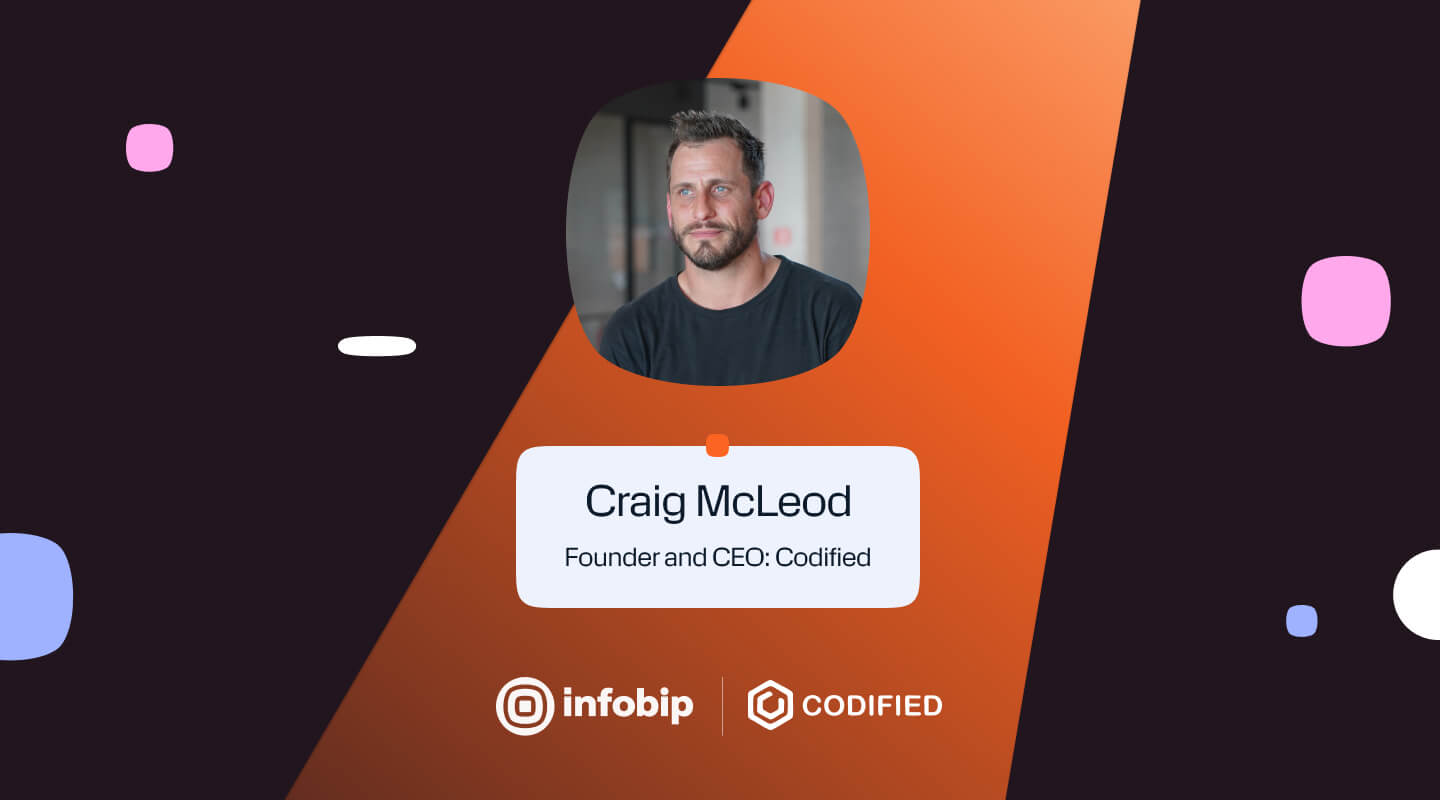
July 22, 2025
Codified: Driving real transformation through conversation
With its roots in customer service and a hands-on, collaborative approach, Codified helps South African enterprises design smarter, conversational experiences built for how people engage.
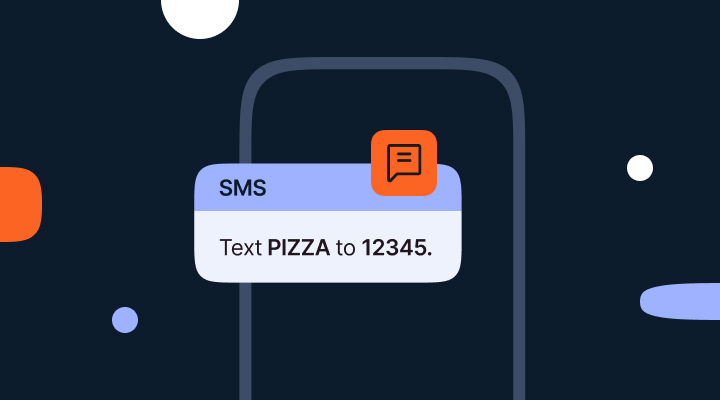
July 22, 2025
The complete guide to SMS keywords: With real examples & tips
Discover how to use SMS keywords to create high-converting text messages. Learn best practices, examples, and tips to improve your SMS marketing strategy.
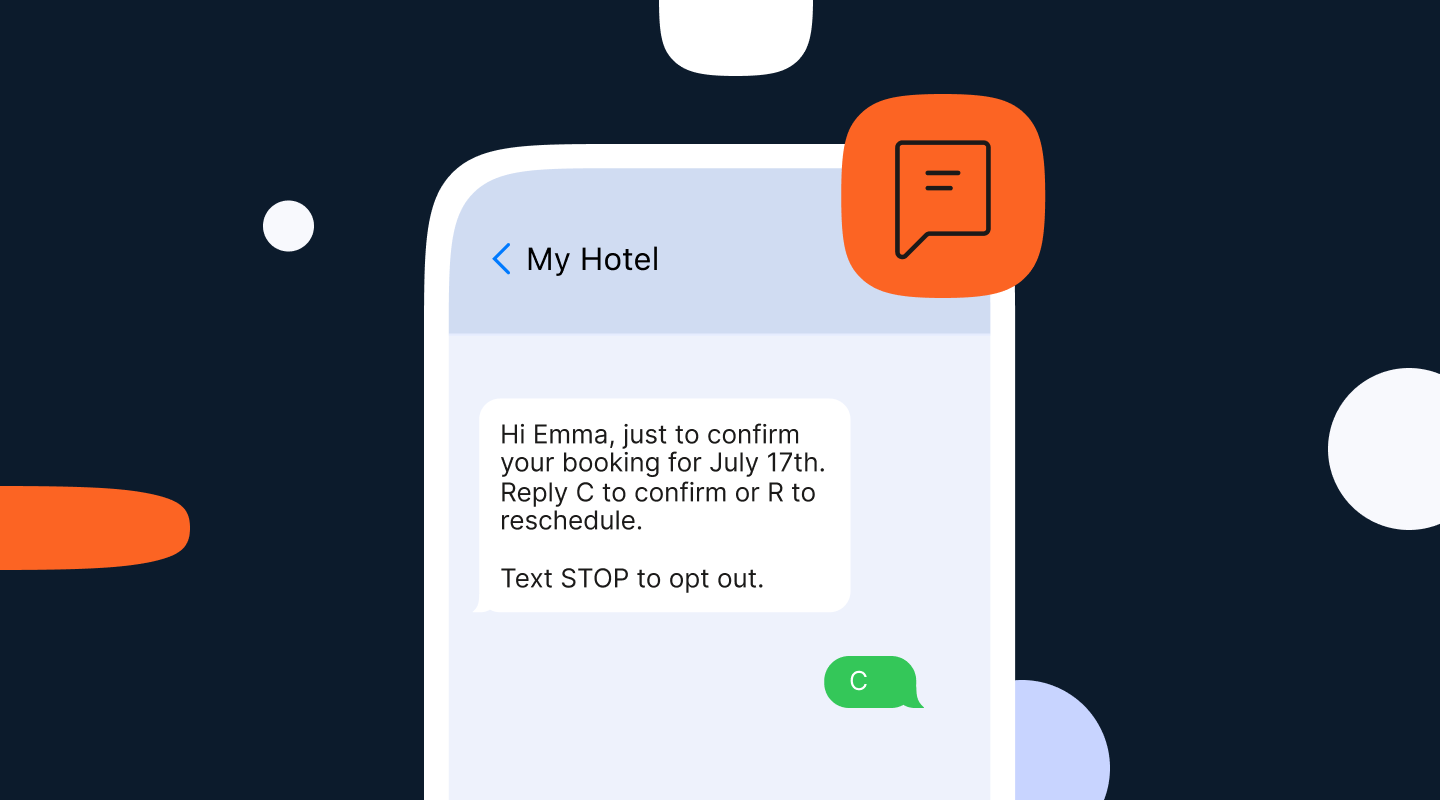
July 21, 2025
Automated text message responses: 15+ examples and best practices (2025)
Learn how to automate text replies with ready-to-use templates, easy setup steps, and smart tips to keep your flows effective.
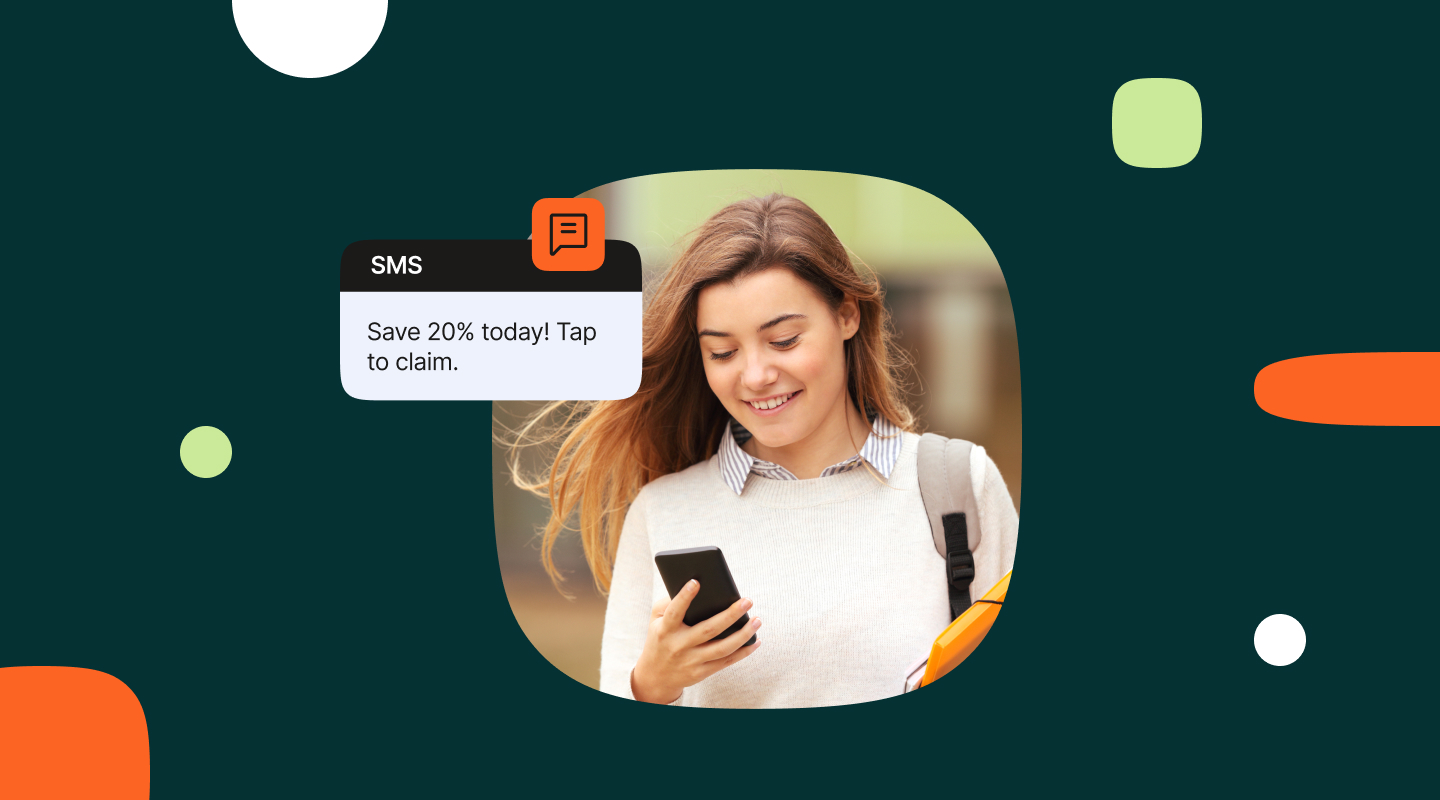
July 21, 2025
How to write SMS marketing copy: 10 tips that drive results
Learn how to write SMS for marketing with 10 expert tips for 2025. Discover best practices for effective, compliant SMS campaigns, including copywriting essentials, timing, personalization, and more.
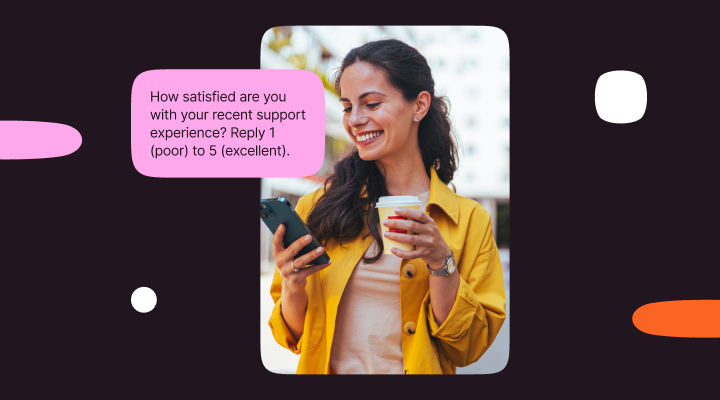
July 18, 2025
How to build professional SMS surveys (tips+examples)
Are you collecting the feedback you need, when it actually matters? SMS surveys are changing how brands capture real-time insights, giving you a direct line to your customer’s experience while it’s fresh, no app downloads, email logins, or delays required.
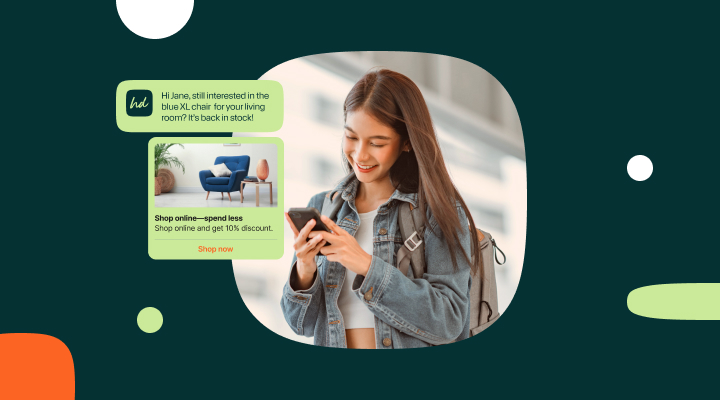
July 18, 2025
Everything you need to know about conversational commerce
Discover everything you need to know about what conversational commerce is and how it will help your business gain a competitive advantage by meeting high expectations.
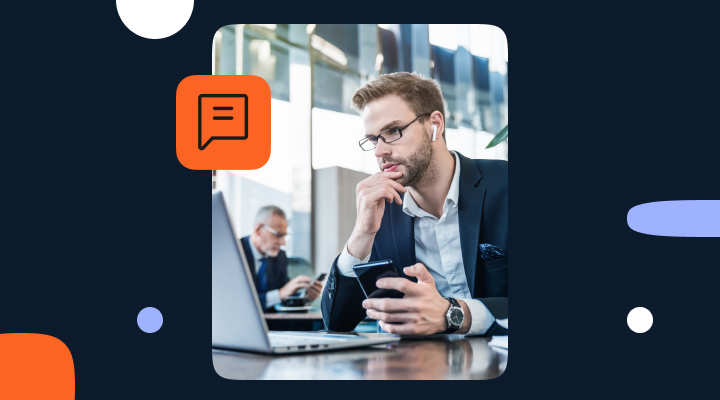
July 17, 2025
SMS terms and conditions: A tool for staying compliant and building trust
A guide to how effective SMS terms and conditions are not just a legal requirement but can be a tool for building customer trust.
{{date_formatted}}
{{title}}
{{excerpt}}